
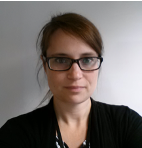
Dominique Perron
Product Owner (PO), Project Manager
Geomatic Expert
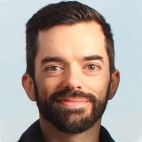
Simon Hallé
Expert in Artificial Intelligence (AI)
Engineering
In 2018, in collaboration with its industry partners, the Government of Canada and the provincial governments of Ontario and Quebec, Thales invested in the ENCQOR 5G project to participate in the development of 5G technology and support the creation of a 5G communications network in Canada. This technology will enable the secure, ultra-high-speed transfer of data to support the evolution of Thales world-class urban transportation solutions, connectivity capabilities and cloud–based Big Data analyses. Today, one of the projects carried out by Thales in this framework is to test and demonstrate real-time data analysis in support of autonomous vehicles, in particular to reduce the autonomous vehicle’s response time to obstacles, even in the harshest conditions such as those experienced in Canada. In an autonomous vehicle, each sensor collects data related to the various functions required for driving. Initially, the Thales research project focuses on perception functions such as vehicle geolocation, recognition and positioning of objects, neighbouring vehicles, obstacles, road tracks, etc. Performing all these functions in real-time requires too much computing power to be executed on the onboard computer found in the vehicle, however powerful it may be. Therefore, the team wishes to test iteratively the possibility of offloading certain functions using edge or even cloud computing.
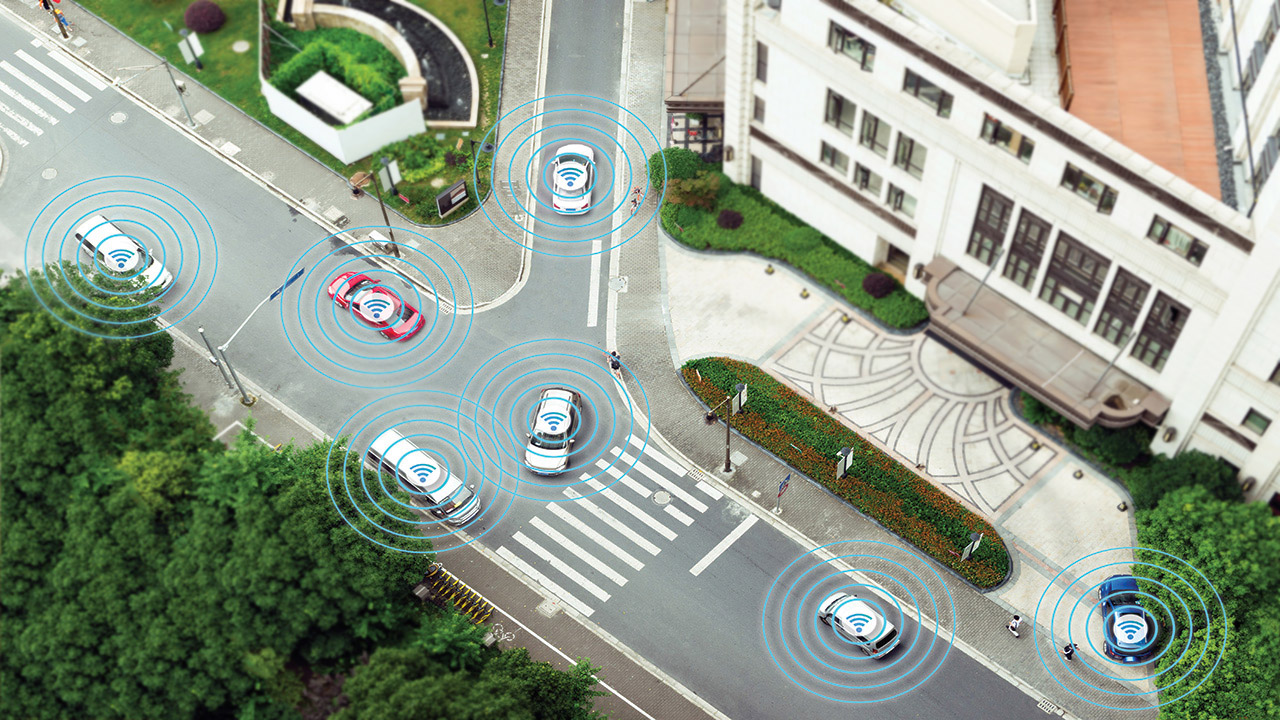
To do this, the first phase of the project is an evaluation of the functions that edge computing could potentially support depending on the data type (volume, frequency) and the required computing time, using theoretical information such as simulation. To date, the results of this work have been very promising. Using data on transmission speed and latency, collected using a 5G phone, the research team was able to validate the actual performance of the technology and train artificial intelligence models. In addition, researchers were able to exploit the collected data to generate new cases in order to broaden its test dataset using synthetic data. Thanks to this work of modeling fictitious environments and “what-if” prediction, the team is able to obtain predictions on the performance of the 5G connected vehicle in non-captured contexts (weather, traffic, obstacles, breakdowns, etc.) to assess the performance and safety of the vehicle in such situations. These results will be validated through the 5G network by integrating sensors typically required to drive an autonomous vehicle (cameras, GPS, IMU, LIDAR, etc.) and by using image-based location services allowing to confirm the GPS position. The next phase of the research will address demonstrations in a “collaborative” framework by allowing the algorithms that have been developed to use data from other vehicles, road infrastructure, or cloud computing services.